Ontologies (Part 1)
By Yuri Chernishov, Head of R&D Center
Introduction
In our world new things appear daily. New knowledge domains that were never thought of just several years ago, appear on a regular basis while old domains disappear, unable to sustain competition. Each knowledge domain is defined by specific knowledge that describes domain objects and their properties. Practical use of this knowledge is maintained by the experts. Even more, professional competencies of the experts are defined by possession of specific knowledge although rapid changes in technological innovation make obtaining wide and deep expertise a challenge. One of the reasons behind this is the huge amount of data generated by each and every subject domain and industry.
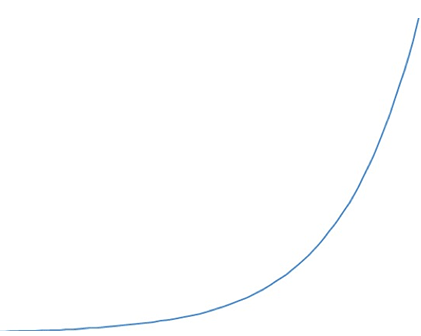
Contemporary observations show that the growth of data amount has become exponential, in other words, the growth rate of data amount depends on the current data amount linearly. The more data there are, the higher becomes the rate of the data amount growth. The importance of this trend can hardly be overestimated – both technologically and psychologically. Enormous amounts create difficulties in data transfer, processing and storage, despite significant increases in hardware performance. Yet, the true challenge lies not in the mere amount of data, but rather in the fact that the data has no structure. Data are provided by different sources, in various formats and at different time periods. In order to store and use these data in practical tasks preprocessing is aimed at making the data structured and converting it into suitable formats. A traditional way to store and use data is based on relational databases where the data are stored in relational tables. However, in many cases use of tabular data is ineffective. As the result, alternative forms such as Knowledge Organization Systems (KOS) have been developed. Their use is based on knowledge graphs.
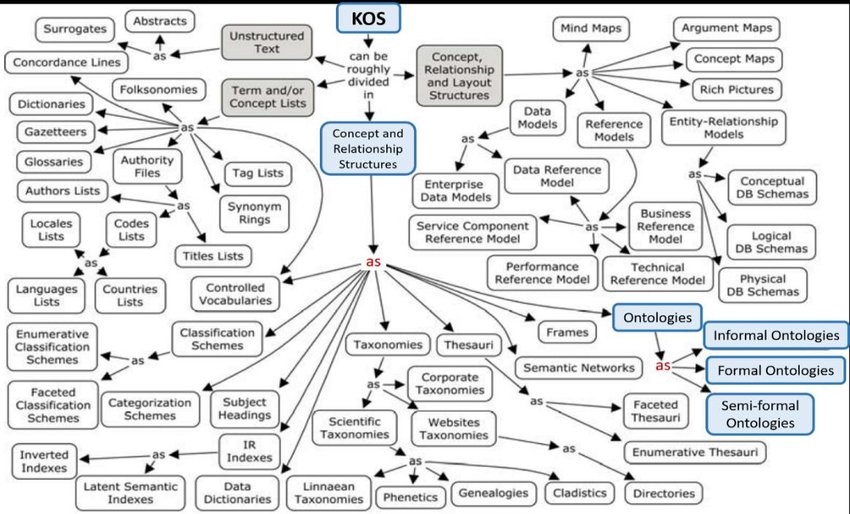
Various structures are used to store knowledge:
- Controlled vocabularies: knowledge arrangement method for subsequent search implemented in subject indexing schemes, subject entries, thesauruses, taxonomies and other KOS.
- Thesauruses: merge terms into groups by a specific property such as resemblance (synonyms).
- Taxonomies: categorized words organized by a hierarchical trait.
- Ontologies: description of formal knowledge from a domain (subject domain) considering existing complex rules and relations between elements that allow automatic knowledge extraction (reasoning).
- Datasets: machine-readable data sets.
Ontologies are developed for Knowledge Organization Systems and are essential in spheres where detecting new facts and identifying hidden relations between components (for example, recommender and expert systems) are imperative. This is an alternative to classic databases where “closed-world assumption” is implemented, in other words, it is assumed that everything that is not included into the database does not exist. In contrast, “open-world assumption” is adopted in ontologes where we assume that if a knowledge base does not include something, it does not mean that it does not exist. It rather means that it has not been described yet.
Knowledge organization systems are widely spread and implemented in many industries. A striking example is a knowledge graph developed to search information in the Internet. It has considerably improved search quality. Some other ontology implementation examples include:
- Banks use knowledge graphs for fraud detection.
- Graphs based on legal documents are usually implemented in consulting.
- Aggregated data based on patient health is used in healthcare, Health Electronic Record.
- Knowledge graphs are implemented in various industries for supply-chain management. In fact one of the main features of the Industry 4.0 is the interaction of cyberphysical systems that leads to automation and demands some form of knowledge management.
- Knowledge bases are often used to manage chat-bots as well as to process complex queries in natural language (for example, asknow service).
- Ontologies are also applied in a wide range of natural language processing tasks: text annotation by means of ontologies, knowledge extraction, NER, Named Entity Linking, Relation Linking, automated new knowledge deduction, reasoning. SemTech solutions see rapid development all around the world as well.
Ontologies are also applied in information security. Installing patches and updating software in order to decrease attack possibility will never provide a 100% protection due to vulnerabilities caused by insecure user behavior and infrastructure configuration, errors in implemented security tool configurations, password configurations and insufficient privileged access control. Protection from zero-day attacks is extremely difficult as well, as no rules exist that would detect this attack type, both recognition and response are supposed to take place on the fly. One way to recognize an attack with an unknown pattern is to use accumulated knowledge and reasoning taking into account all available information on current events. Such knowledge can be stored by means of ontologies where the data about correlations between various entities are stored.
What are ontologies?
Ontology is mathematically based on the so called description logic (a branch of mathematics) that assumes that any information expressed in a natural language can be represented as triplet series.
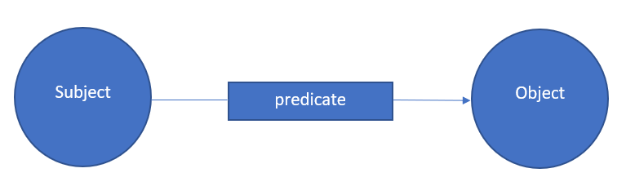
A passage from an English nursery rhyme “The House That Jack Built”.
…
This is the rat,
That ate the malt
That lay in the house that Jack built.
…
The relations between different entities described in the rhyme can be represented as an ontology.
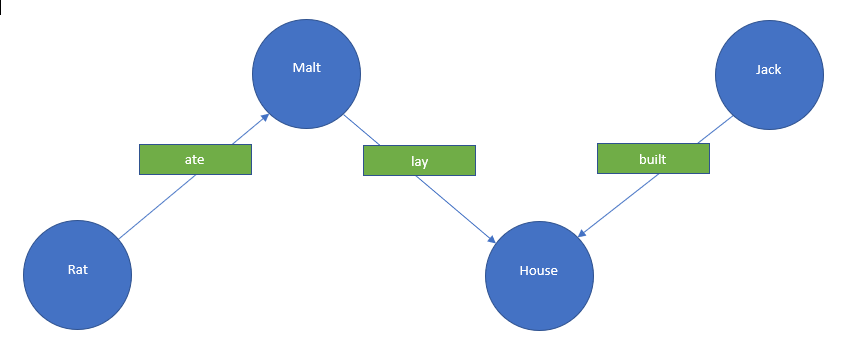
The ontology is represented as a graph where the nodes are entities and the arches are relations between the entities. It is deemed that any statement in natural language can be represented as simple sentences and entities as well as relations between these entities can be extracted from the sentences. There are two main tools: RDF (Resource Description Framework) or OWL (Ontology Web Language). One of the OWL features is support for descriptions for the logical rules for the data. Ontologies (in contrast to standard databases) help find hidden data. Standard ontologies are applied when a search for specific information is required and knowledge bases are intended to identify new knowledge, for instance, in decision support systems (expert systems).
RDF store examples: Virtuoso, 4store, stardog.
Ontology is particularly useful when a detailed and thorough description of the relations between the components is provided by means of a mathematical tool of descriptive logic. For example, properties can be assigned to relations (functional, transitive, reflexive). As a result, facts are automatically extracted from ontologies; this process is defined as reasoning. There is a variety of reasoning algorithms based on graphs. Here are some examples of application options: refining object characteristics and extraction of a unique object from a set of similar objects, search for similar objects, “text understanding” and text classification, assistance in NLP tasks (NER, Relation Extraction), root cause analysis, pattern detection. There is a wide variety of tools that support reasoning such as as IBM Watson, Wolfram Alpha. However, the most popular editor is Protégé.
Ontologies are usually created manually by professionals. Though, there also are examples of automatic ontology creation based on existing knowledge bases. Open-source knowledge graphs (at the end of 2021, according to data provided by https://lod-cloud.net/:
- DBpedia
- Yago + wordnet.princeton.edu
- WikiData
- Open-source knowledge base including object and industrial knowledge bases, for example, healthcare: BioPortal, Bio2RDF, PubMed
The tools above provide various methods for operation with ontologies, however as the most popular tool is Protégé, we shall base our further discussion on its logic and features.
Working with Protégé
Installation
Protege is designed by Stanford University to develop, edit and use ontologies. The software is free and can be downloaded from https://protege.stanford.edu/products.php; web version is also available on https://webprotege.stanford.edu/ and an archive file is provided on the official page for using it on a local computer. It is important to account for the operating system and processor architecture (64-bit or 32-bit). The latest Protege version (at moment of writing this) is 5.5, however, legacy 32-bit operating systems would require older Protégé versions, such as Protégé 4.3.
Extract Protégé.exe file to any folder and start it. Now you can create ontologoes. But it is just the beginning of a long and arduous journey, but an interesting one.
Project creation
When the program starts the following window opens.
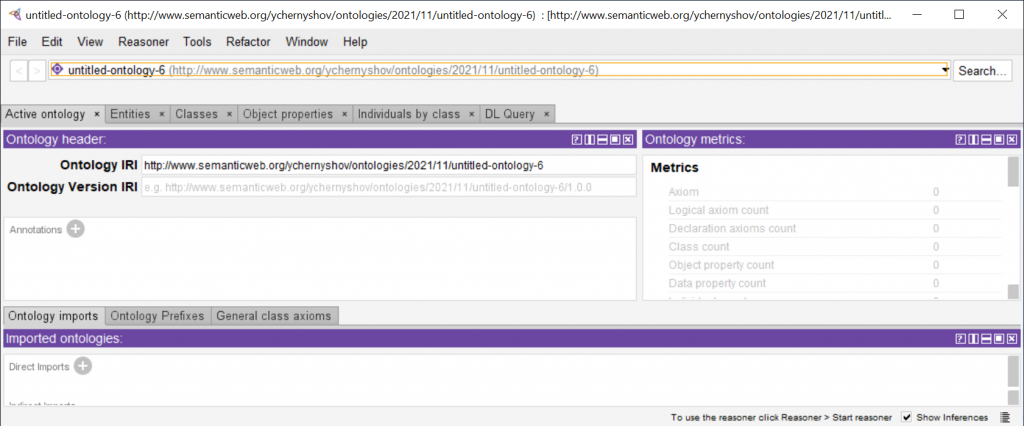
Everything is in English and Java here, but English is actually enough for understanding.
Each project has a unique identifier – IRI (Internationalized Resioure Identifier).
Protégé allows to record triplets represented as “subject-predicate-object”.
The Entities section allows describing subjects and objects.
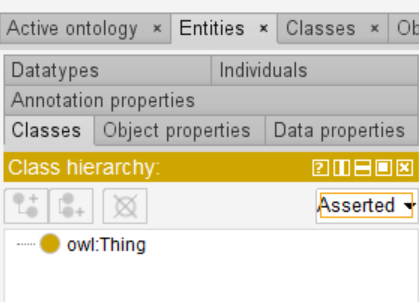
Important tabs in the section:
- Class instances (Individuals). The same class objects as in object-oriented programming. For example, the “Server” class, “prod-serv-002” object.
- Properties (object properties or data properties). Similar to class properties in object-oriented programming. However, in ontologies properties are independent and can be separated from the class (unlike object-oriented programming).
Various properties can be assigned to predicates, i.e.:
- Functional
- Reverse
- Transitive
Reasoner can be applied to the described ontology. It makes offers based on the obtained facts (which can be accepted or rejected).
Ontologies can be saved as an owl file and look exactly like a typical xml file if opened in a text editor.
It will be easier to explain these concepts on examples.
Practical tasks for Protégé
Personnel access to rooms
Let’s imagine a situation in which we need to track the location of each employee. We know that:
- Johns does not have access to the server room and the room 101.
- Hansen does not have access to the 101 room.
- Smith does not have access to the document storage room.
There are three employees (Johns, Hansen, Smith) and three rooms (the server room, room 101 and the document storage room). For a clear-cut solution, ontology component properties must be strictly defined, for instance, establishing the fact that there are no other employees and rooms and that one employee has access to only one room otherwise it will be impossible to logically solve the task. If strict restrictions are set up, task solution will be trivial. The first condition implies that Johns has access to the document storage room (since the server room and the room 101 are excluded); Hansen (the server room and the 101 room are left) has access to the server room and Smith is left with the room 101. Now it will be easier to check operation results of the Protégé reasoner, which was trusted to solve the problem.
Start Protégé editor.

Now we can start creating an ontology for the task in the main window. At first, let’s create two classes: “Employees” and “Rooms”.
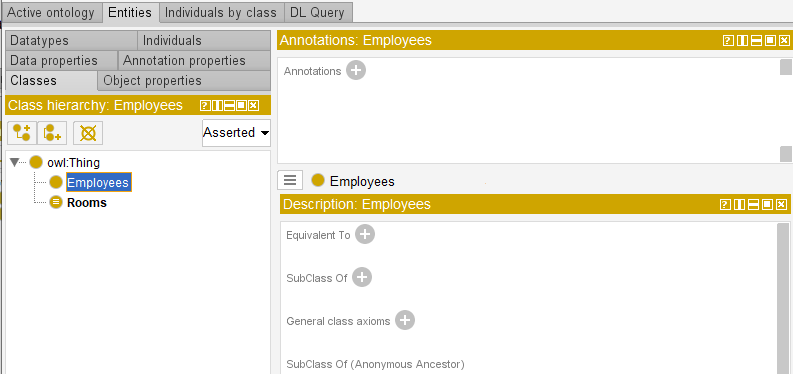
Let’s create instances for these classes.
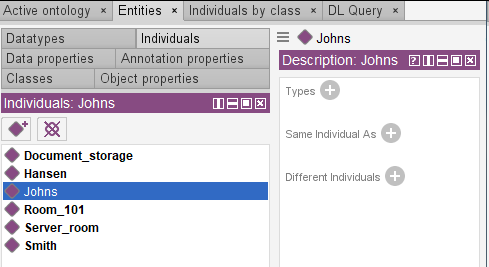
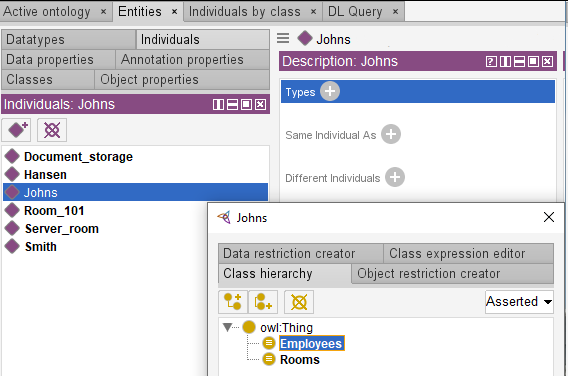
Let’s repeat this procedure for the remaining employees (Hansen and Smith) and the rooms.
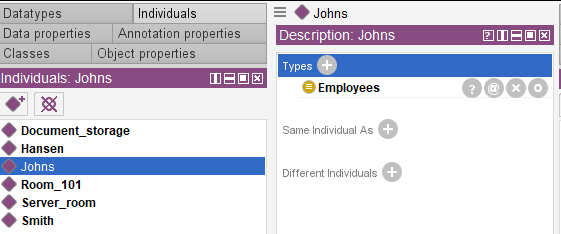
Now object property “inside_room” can be created. It will connect Employees and Rooms. When object property is created, entities related by the predicate should be specified. In our case they are Employees (Domain) and Rooms (Ranges). Employees can be in any room and a room can accommodate any number of employees. The property is functional and acts from the Employees domain to the Room range. Based on principles of biology and physics, one employee can be only at one room. This fact must also be taken into account when “inside-room” property is determined. Functional and Inverse parameters should be assigned to this property; as a result binary relation “one-for-one” will be formed.
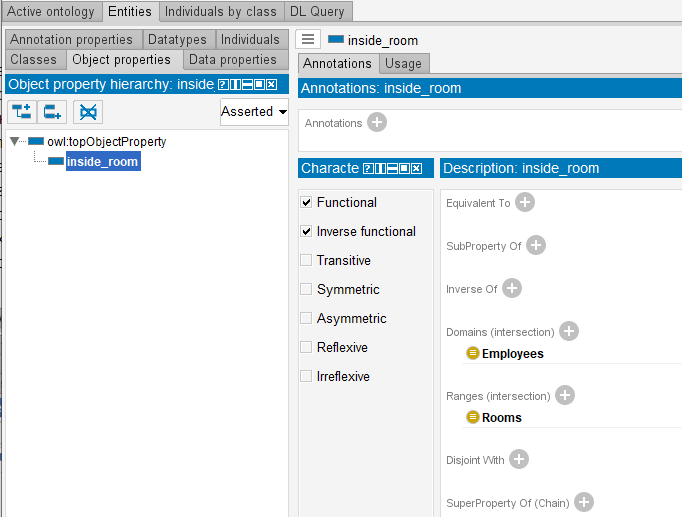
Let’s add known information on employee location to our ontology.
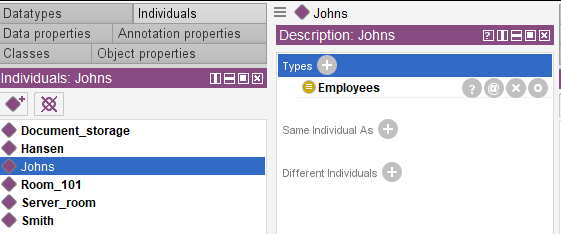
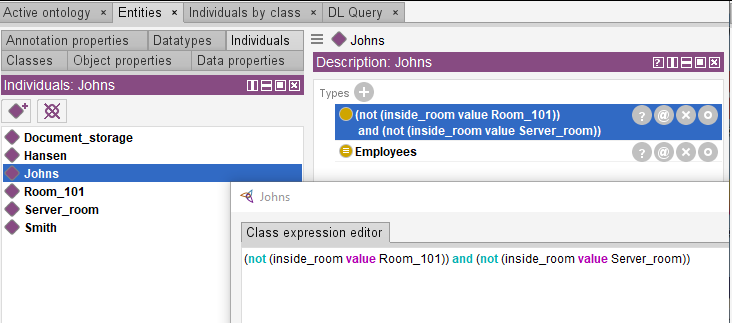
Let’s add similar information on Hansen and Smith using logical predicates “not” and “and”.
We can try to start a Reasoner.
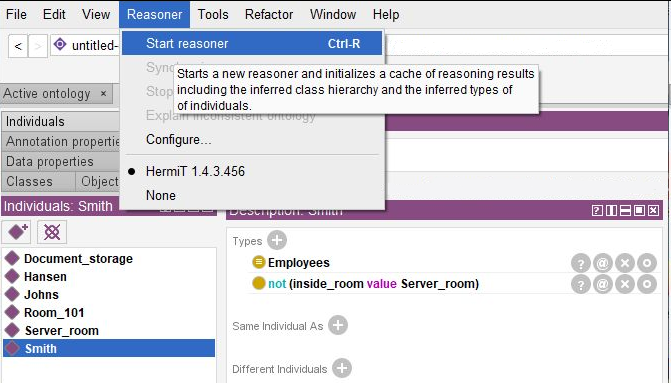
However, new objects (employees and rooms) still can be added to the current statement according to the open world hypotesis therefore no significant facts can be extracted. To conclusively solve the task referred to employee location detection, the reasoner requires strictly defined properties for “Employees” and “Rooms” classes.
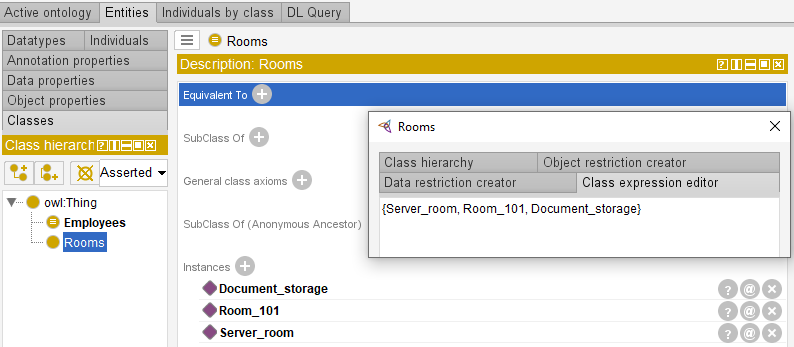
To establish one-to-one relations between the ontology entities it is required to specify that each employee must be at least in one room.
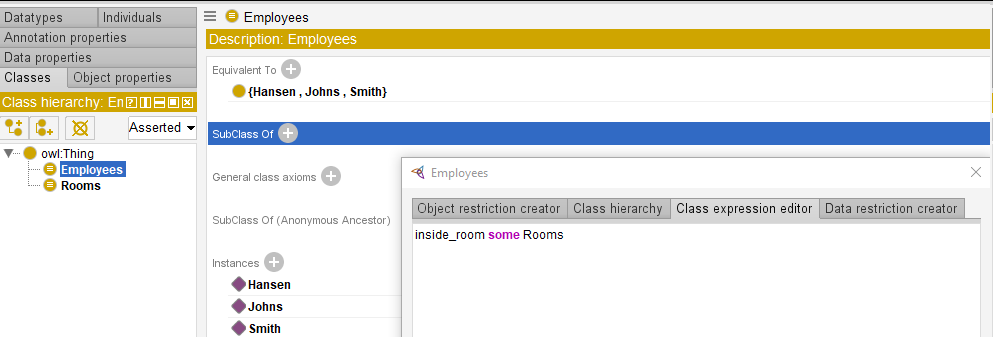
Starting (or synchronizing) the reasoner will provide information on the employee exact location in a certain room. For example, we have established that Johns is in the document storage room.
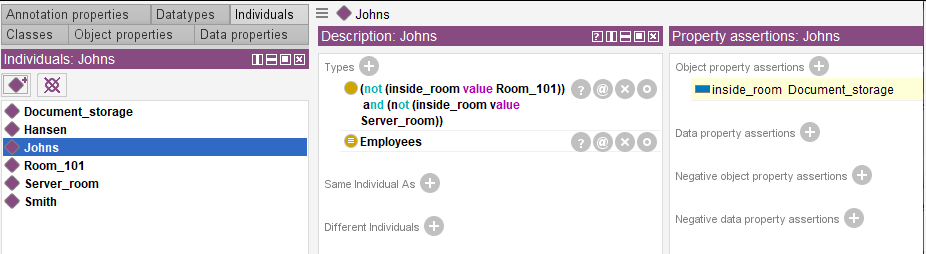
The selected ontology can be saved e.g. in owl format.
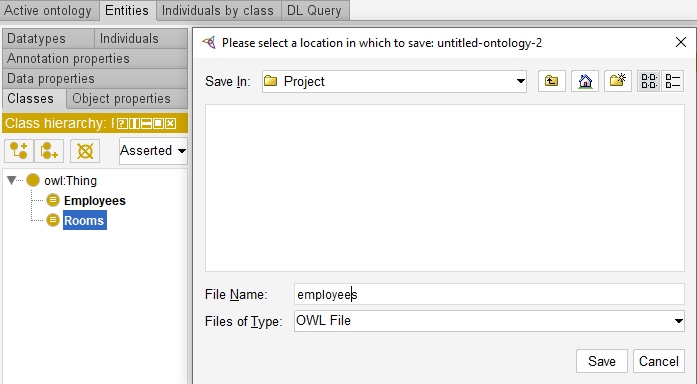
The obtained .owl file can be opened in any standard text editor. However, information is perceived better when represented in a graph and Protégé provides such option.
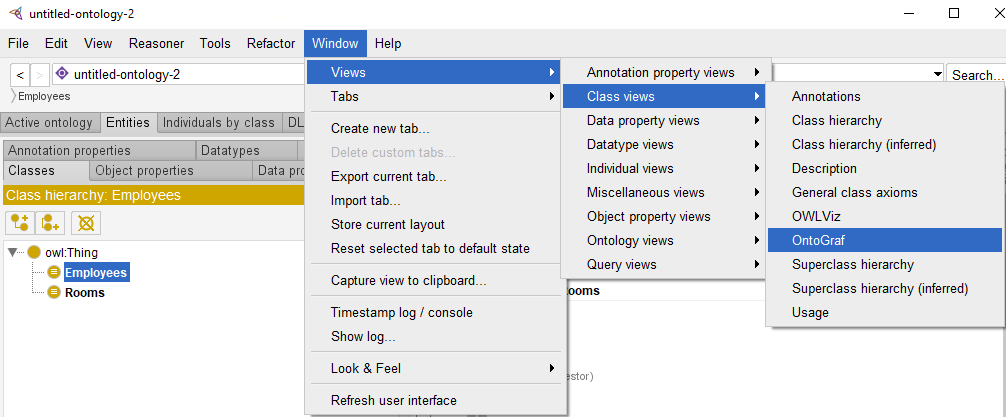
As a result, an informative visualization is generated.
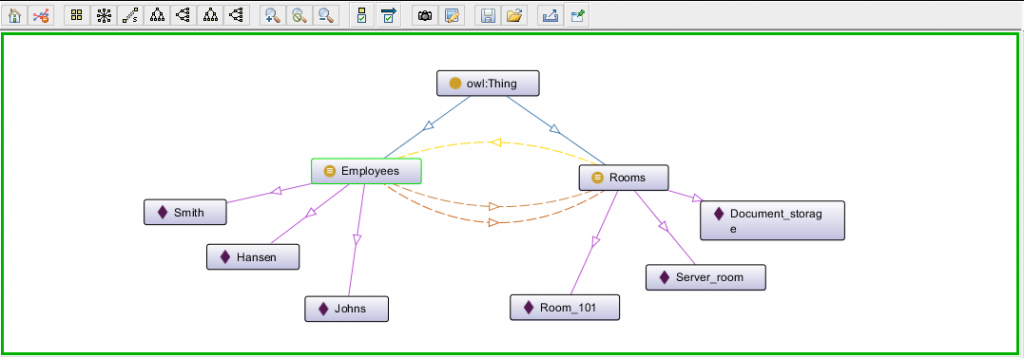
As the described problem is quite simple one and can be solved by unsophisticated logical reasoning. The problem that we are going to discuss next is more complicated and only few people can solve it in their minds.
Einstein’s Riddle
Problem statement
An Einstein’s Riddle is a well-known logical puzzle. It consists of 15 clues meant to help in finding answers to the questions of who drinks water and who owns a zebra.
The original riddle text is as follows:
- There are five houses.
- The Englishman lives in the red house.
- The Spaniard owns a dog.
- Coffee is drunk in the green house.
- The Ukrainian drinks tea.
- The green house is immediately to the right of the ivory house.
- The Old Gold smoker owns snails.
- Kools are smoked in the yellow house.
- Milk is drunk in the middle house.
- The Norwegian lives in the first house.
- The man who smokes Chesterfields lives in the house next to the man with the fox.
- Kools are smoked in the house next to the house where the horse is kept.
- The Lucky Strike smoker drinks orange juice.
- The Japanese smokes Parliaments.
- The Norwegian lives next to the blue house.
Who drinks water? Who owns a zebra?
In the interest of clarity, it must be added that each of the five houses is painted a different color, and their inhabitants represent different nations, own different pets, drink different beverages and smoke different brands of American cigarettes. One other thing: in statement 6, right means your right.
Solution
The first stage includes determining classes. There are five classes: “Houses”, “Men”, “Animals”, “Drinks” and “Cigarettes”. The “Houses” class has two features which are house number and color therefore it is reasonable to create a new class – “Colors”.
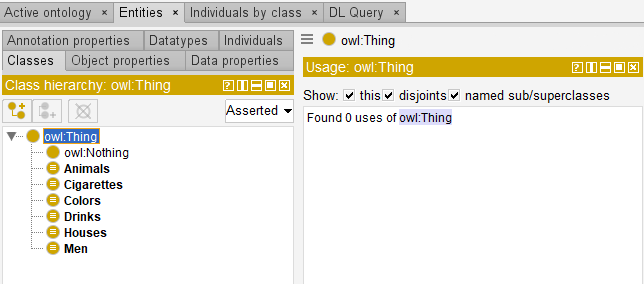
The next step is to create class instances (objects).
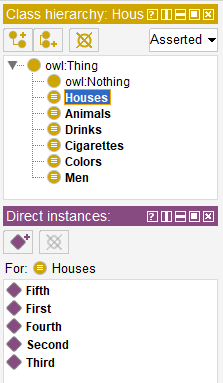
The rest five classes are subject to the analogous procedure.
Then properties for objects and object characteristics are to be created. Six properties (predicates) will be created in the “Object Properties” section: “live_in”, “has_color”, “has_animal”, “smokes_cigarettes”, «located_right_of», “drink_a_drink”. Entities related by predicates and their characteristics are to be specified in the process of predicate creation.
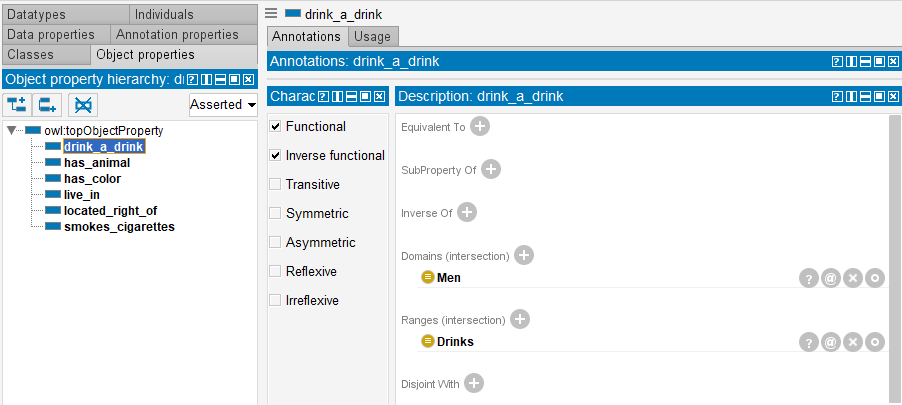
Let’s run the same procedure for the other predicates.
Functional and Inverse functional parameters are assigned to all six properties. It is a binary “one-for-one” relation.
Now it is time to add all known facts about entities to the ontology. The first fact is that the houses are in 1-5 order. For this purpose, we will specify that the “First” object is to the right of the empty set and that the “Fifth” object it is to the right of the forth house and to the left of the empty set.
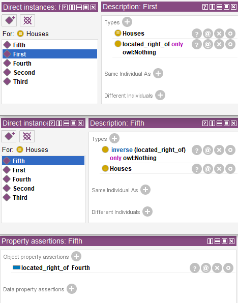
For the “Second”, “Third” and “Fourth” objects we should specify that they are to the right of the previous one in the “Object property assertions”.
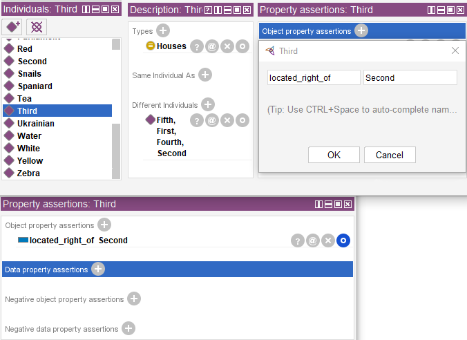
It is also required to add information from simpler statements represented as triplets such as “Spaniard owns a dog”.
Next step is adding information contained in the riddle statements. Though, by the first look, this information does not help in searching for the answers required.
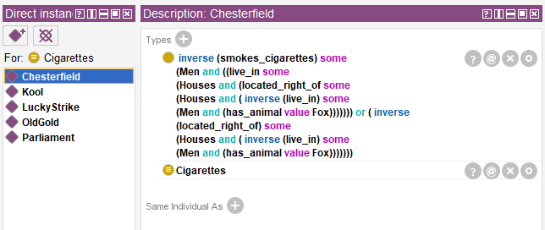
In the example above, service word inverse makes a cigarette brand the statement subject because Chesterfield is smoked by the man and not vice versa.
The information from other statements will be added in the same way. If we try and start the reasoner now, no significant facts can be extracted because all classes are open in the current statement and an option to add new objects is preserved. The reasoner will be able to solve the problem only if the classes are strictly determined.
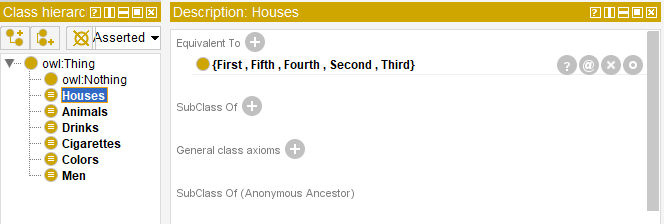
The other five classes must be determined in the same way. To define the ontology the fact that each man definitely leaves in a house, drinks a drink, owns a pet and smokes the cigarettes of a certain brand must be indicated.
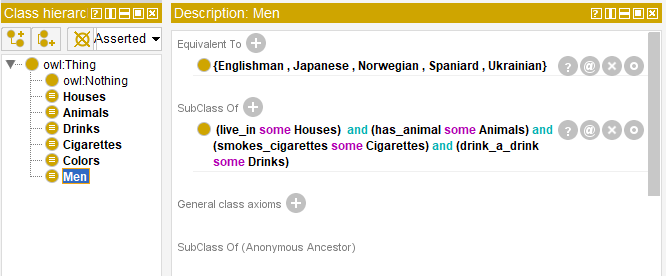
Starting the reasoner will provide us answers to the questions. Now we know that the Norwegian drinks water and the Japanese owns zebra.
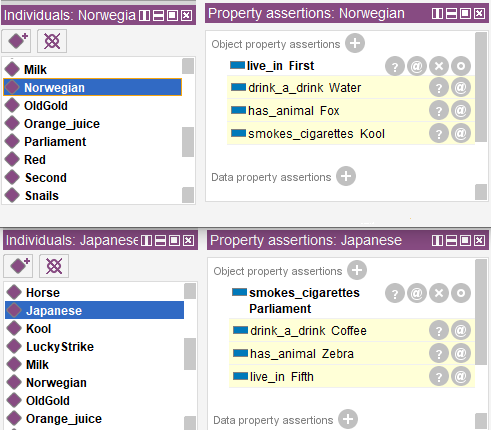
Obviously, well-described ontologies can help solve difficult problems, however, it is required to meticulously describe properties of an ontology components, entities and predicates.
Conclusion
It is important to realise that ontologies implementation is not always efficient in solving problems. The solution method is to be determined based on subject domain particularities.
Various knowledge bases have already been created in information security such as MITRE ATT&CK and SHIELD, CVE, CAPEC. They are implemented in incident analysis and response, investigations and vulnerabilities detection. But let this be the topic of the next article.